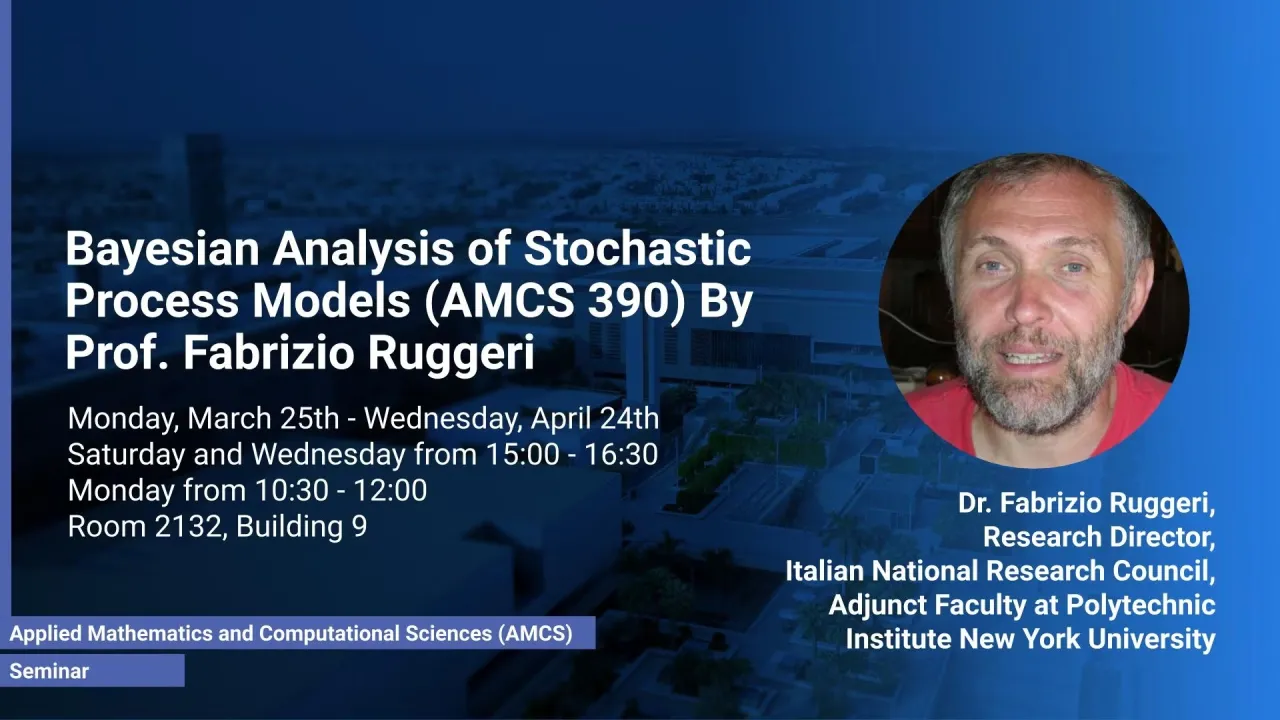
Course AMCS: Bayesian Analysis of Stochastic Process Models
The student will be introduced to Bayesian modeling in selected, but relevant, stochastic processes and their applications: Markov chains, Poisson processes, reliability, and queues. The use of real examples will be helpful in understanding why and how to perform a Bayesian analysis.
About
Instructor: Dr. Fabrizio Ruggeri
Course material available at: link
Class Schedule:
At the Room 2132 in Building 9, Saturday and Wednesday from 15:00 PM to 16:30 PM and Monday from 10:30 AM to 12:00 PM. Starts on Monday, March, 25th and ends on Wednesday April, 24th.
Course Objectives:
The student will be introduced to Bayesian modeling in selected, but relevant, stochastic processes and their applications: Markov chains, Poisson processes, reliability, and queues. The use of real examples will be helpful in understanding why and how to perform a Bayesian analysis. Students will be asked to analyze real data, from the elicitation of priors and modeling to (numerical) computation of estimates and forecasts and interpretation of findings.
Course Outline:
Introduction to Bayesian Analysis
Inference and prediction for discrete-time and continuous-time Markov chains
Inference for Homogeneous and Nonhomogeneous Poisson processes
Inference for stochastic models for repairable and non-repairable systems
Inference and decision problems in queueing systems (M/M/1 queues, non-Markovian systems)