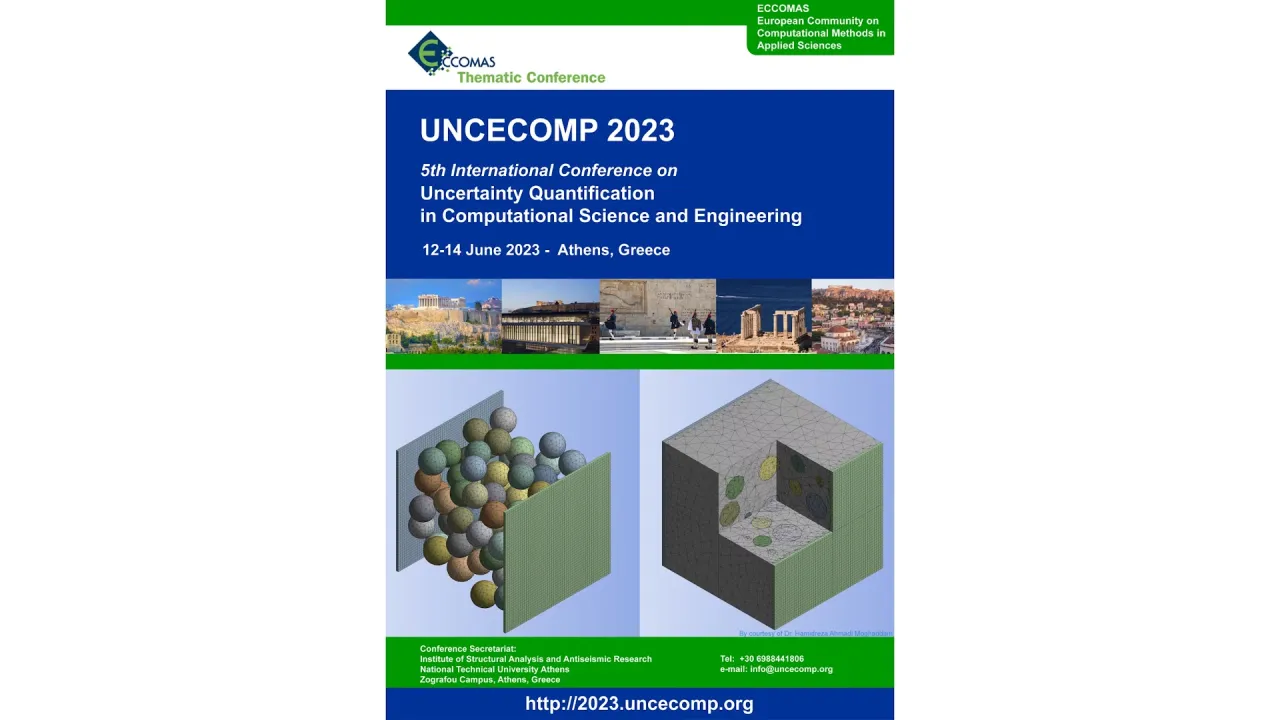
Post-Doc André Carlon participation at UNCECOMP2023 in Athens
About
A postdoctoral fellow of our group Dr. Andre Carlon participated in the recently concluded 5th international conference on Uncertainty Quantification in Computational Science and Engineering and presented a talk on Adaptive double-loop Monte Carlo gradient estimators for Bayesian optimal experimental design. The conference held at Athens, Greece between 12-14 June, 2023.
Abstract:
Designing experiments is a challenging task. Models of experiments can be used to improve their design and maximize informativeness. In Bayesian Optimal Experimental Design (OED) with non-linear models, one uses the Expected Information Gain (EIG) of an experiment to measure its goodness, aiming at the set-up that provides more information about the parameters of interest. If the EIG is continuous and smooth with respect to the experiment's design parameters, gradient-based methods can converge to local maxima. We investigate two estimators for the EIG's gradient, the double-loop Monte Carlo (DLMC) and its Laplace-based importance sampling variant (DLMCIS). Under assumptions of local concavity and smoothness of the EIG, we provide local convergence guarantees in the $L^2$ sense for a Stochastic Gradient Descent (SGD) method using the DLMC and DLMCIS estimators. To obtain SGD convergence, we adaptively control the statistical error and bias of the gradient estimators, including the bias of the experiment model approximation. We prove that SGD with the adaptive DLMC or DLMCIS gradient estimators finds an experimental set-up with a gradient norm less than $TOL$ with computational work $O(TOL^{-3 - \gamma / \eta})$, where $\eta$ is the convergence rate of the error of the model approximation using some parameter $h$ and $\gamma$ is the rate at which the cost increases with $h$. To verify our analysis numerically, we present numerical examples using an analytical experiment model and one based on a finite element method approximation.