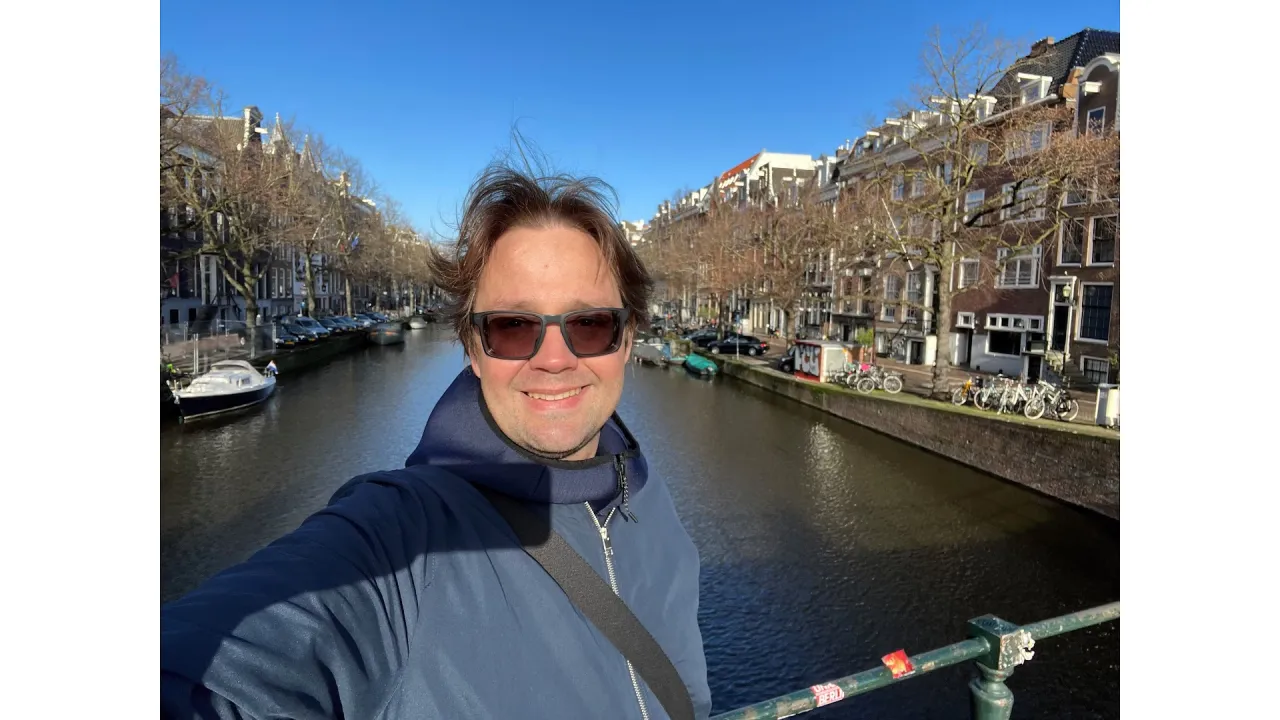
Post-Doc Aku Jakko Kammonen participation at Siam Conference on Computational Science and Engineering (CSE23)
About
A postdoctoral fellow of our group Dr. Aku Jaakko Kammonen participated in the recently concluded Conference on Computational Science and Engineering (CSE23) and presented a talk on Adaptive random Fourier features based on Metropolis sampling. The Conference held at Amsterdam, Netherlands, between February 26 - March 3, 2023.
Abstract:
The supervised learning problem to approximate a function $f:\mathbb R^d\to\mathbb R$ by a neural network approximation $\mathbb{R}^d\ni x\mapsto\sum_{k=1}^K\hat\beta_k e^{{\mathrm{i}}\omega_k\cdot x}$ with one hidden layer is studied as a random Fourier features algorithm.
Here the mean square loss problem can be solved easily, since it is convex in the amplitude parameters $\hat\beta_k$ given a density $p:\mathbb R^d\to [0,\infty)$ for independent frequencies $\omega_k$. It is also well known that the corresponding generalization error is bounded by $K^{-1}\Vert \vert \hat f\vert ^2/((2\pi)^dp)\Vert _{L^1(\mathbb R^d)} $, where $\hat f$ is the Fourier transform of $f$. In my talk I will first show how the constant $\Vert \vert \hat f\vert ^2/((2\pi)^dp)\Vert _{L^1(\mathbb R^d)}$ can be minimized by optimally choosing the density $p$ and then how to approximately sample from this density, only using the data and certain adaptive Metropolis steps. I will also show results with other activation functions.
Kammonen, Aku and Kiessling, Jonas and Plechac, Petr and Sandberg, Mattias and Szepessy, Anders. Adaptive random Fourier features with Metropolis sampling. Foundations of Data Science, 2020.
Kammonen, Aku and Kiessling, Jonas and Plechac, Petr and Sandberg, Mattias and Szepessy, Anders and Tempone, Raul. Smaller generalization error derived for a deep residual neural network compared with shallow networks. IMA Journal of Numerical Analysis, 2022.