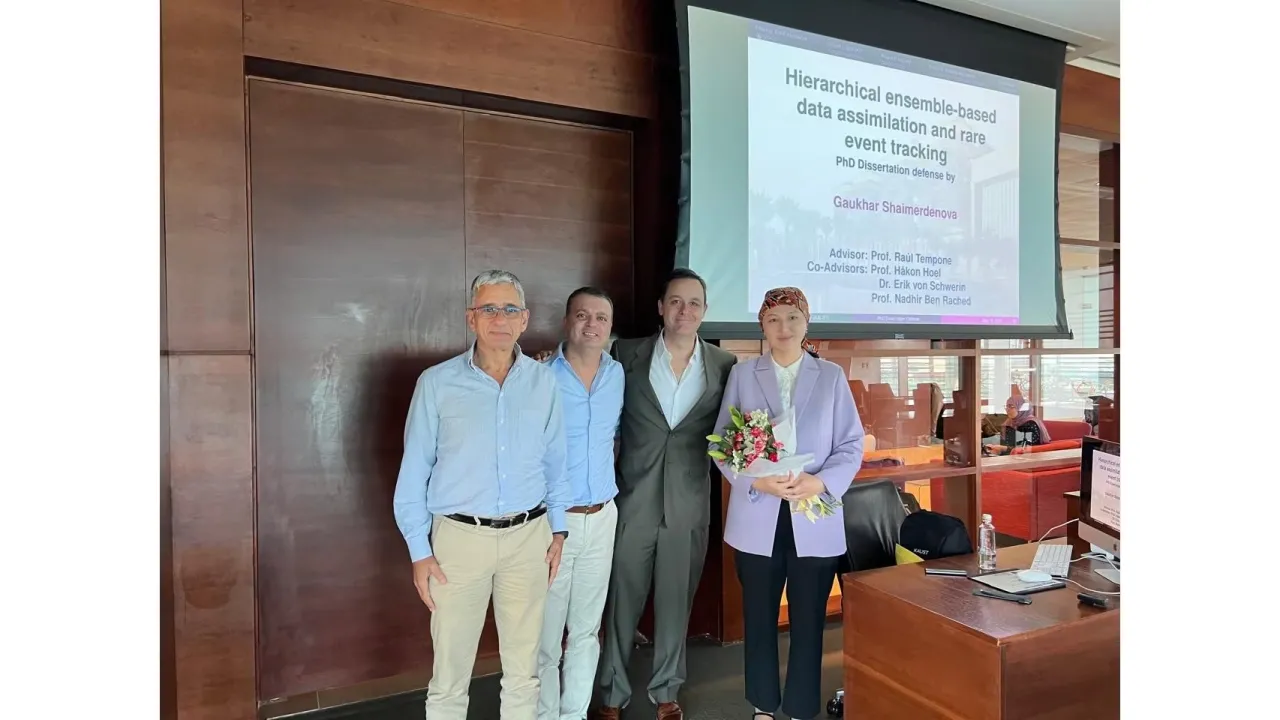
Gaukhar Shaimerdenova succesfully defended his PhD Thesis
About
On May 15, 2024, Gaukhar Shaimerdenova successfully defended her Ph.D. thesis entitled "Hierarchical ensemble-based data assimilation and rare event tracking".
Committee chairperson: Prof. Raúl Tempone, AMCS, KAUST.
External examiner: Prof. Sumeetpal Singh, Tibra Foundation Chair in Mathematical Sciences, School of Mathematics, University of Wollongong.
Committee members:
Prof. Omar Knio, AMCS, KAUST,
Prof. Ibrahim Hoteit, PSE, KAUST,
Prof. Håkon Hoel, University of Oslo,
Prof. Nadhir Ben Rached, University of Leeds.
Abstract:
This thesis consists of three papers considering, in general, two challenges: the minimization of computational cost in the ensemble Kalman filtering (EnKF) method and the problem of tracking a rare event within the framework of the EnKF.
In Paper 1, we present a novel approach to combine the multilevel Monte Carlo methodology with the EnKF to reduce the computational cost of the latter in discrete-time settings with finite-dimensional state spaces and linear observations perturbed by additive Gaussian noise. The new multilevel EnKF (MLEnKF) is constructed as a hierarchy of independent samples of the EnKF, which is fundamentally distinct from the original version previously introduced in the literature and adaptable to extensions into the multi-index Monte Carlo. Compared to the original method, we can establish improved asymptotic efficiency results for the new MLEnKF in specific cases under slightly more rigorous regularity assumptions. In general, thorough theoretical examination and numerical illustrations demonstrate that the new MLEnKF exhibits enhanced computational efficiency compared to the standard EnKF approach, given suitable regularity conditions.
In Paper 2, we explore a further extension of the MLEnKF in the same problem setting introduced in Paper 1 to a more efficient multi-index EnKF (MIEnKF) based on the multi-index Monte Carlo methodology. Compared to pairwise coupling in MLEnKF, the MIEnKF employs a four-coupling of EnKF estimators interconnected not only with numerical time discretization but also with the EnKF ensemble size, resulting in a more substantial reduction of variance. These efficiency enhancements are numerically validated across a set of test scenarios.
In Paper 3, we consider the challenge of tracking a rare event within the context of EnKF with the same filtering problem setting as in Paper 1 and Paper 2. In particular, we consider a low-probability event exceeding a threshold value for a time-varying quantity of interest linked to a stochastic differential equation. Between two EnKF observation time instances, we apply importance sampling (IS) techniques with respect to the initial condition and the Wiener process to be able to monitor the occurrences of rare events. The optimal IS adjustments in the proposed strategies are derived from solving a second-order partial differential equation in a one-dimensional setting. For situations involving multidimensions, the Markovian projection technique is employed. The introduced concepts are assessed through two illustrative examples, revealing significant reductions in variance compared to the conventional Monte Carlo method.
Biography:
Gaukhar Shaimerdenova is a member of the stochastic Numerics group (STOCHNUM) at KAUST, supervised by Professor Raul Tempone. She obtained a Bachelor's degree in Mathematics at Eurasian National University, Kazakhstan, and a Master's degree in Financial Mathematics at University College London, UK. Her research interests include Stochastic Differential Equations, Numerical Analysis, Data Assimilation, Multilevel/Multi-index Monte Carlo methods, Ensemble Kalman Filtering and Computational Finance.