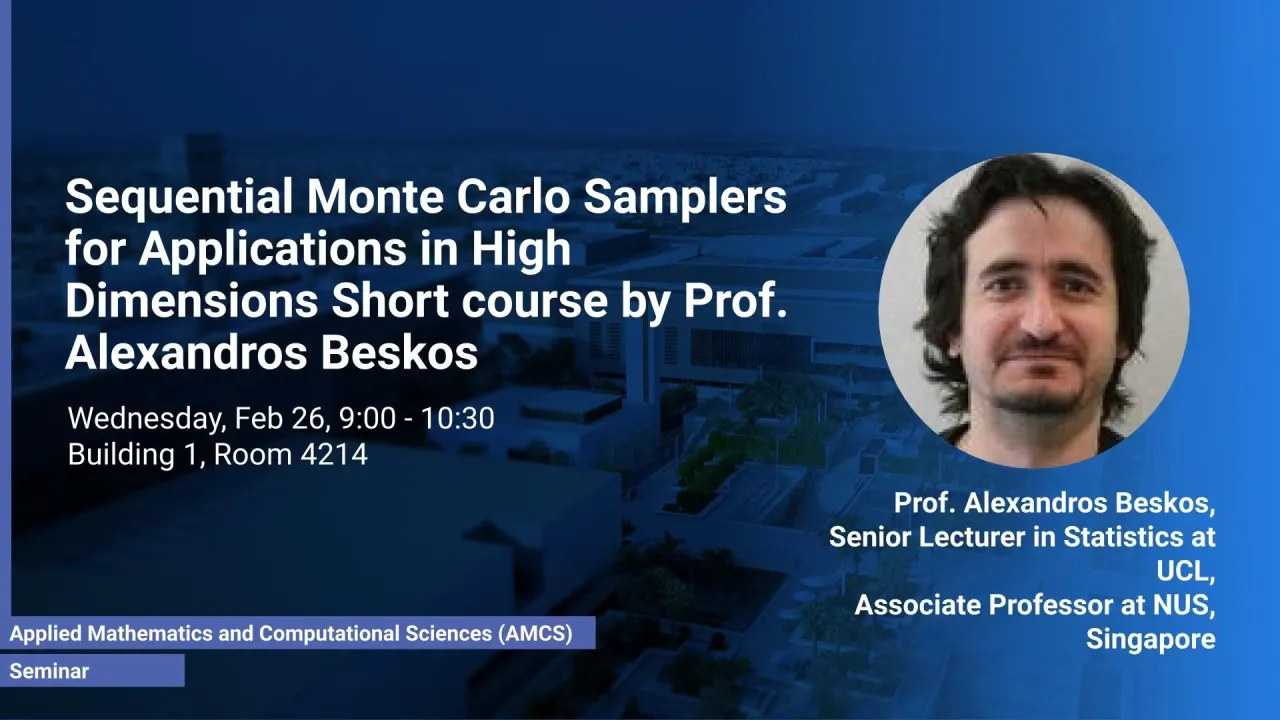
Sequential Monte Carlo Samplers for Applications in High Dimensions Short course By Prof. Alexandros Beskos (Associate Professor at NUS, Singapore)
B1 R4214
Sequential Monte Carlo (SMC) methods are nowadays routinely applied in a variety of complex applications: hidden Markov models, dynamical systems, target tracking, control problems, just to name a few. Whereas SMC methods have been greatly refined in the last decades and are now much better understood, they are still known to suffer from the curse of dimensionality: algorithms can sometimes break down exponentially fast with the dimension of the state space. As a consequence, practitioners in high-dimensional Data Assimilation applications in atmospheric sciences, oceanography and elsewhere will typically use 3D-Var or Kalman-filter-type approximations that will provide biased estimates in the presence of non-linear model dynamics.
Overview
Abstract
Sequential Monte Carlo Samplers for Applications in High Dimensions.
Sequential Monte Carlo (SMC) methods are nowadays routinely applied in a variety of complex applications: hidden Markov models, dynamical systems, target tracking, control problems, just to name a few. Whereas SMC methods have been greatly refined in the last decades and are now much better understood, they are still known to suffer from the curse of dimensionality: algorithms can sometimes break down exponentially fast with the dimension of the state space. As a consequence, practitioners in high-dimensional Data Assimilation applications in atmospheric sciences, oceanography and elsewhere will typically use 3D-Var or Kalman-filter-type approximations that will provide biased estimates in the presence of non-linear model dynamics. The talk will concentrate on a class of SMC algorithms and will look at ways to reduce the cost of the algorithms as a function of the dimension of the state space. Explicit asymptotic results will clarify the effect of the dimension at the properties of the algorithm and could provide a platform for algorithmic optimisation in high dimensions. Applications will be shown in the context of Data Assimilation, in a problem where the objective is to target the posterior distribution of the initial condition of the Navier-Stokes equation given a Gaussian prior and noisy observations at different instances and locations of the spatial field. The dimension of the signal is in theory infinite-dimensional - in practice 64x64 or more depending on the resolution – thus posing great challenges for the development and efficiency of SMC methods.
Brief Biography
2013-Today: Senior Lecturer in Statistics at UCL and Associate Professor at NUS.
2008-2013: Lecturer in Statistics at UCL.
2005-2008: Post-Doc in Mathematics Institute and Department of Statistics, at University of Warwick.
2002-2005: PhD under the supervision of Professor Gareth Roberts
Refreshments: Available @ 08:45 am.