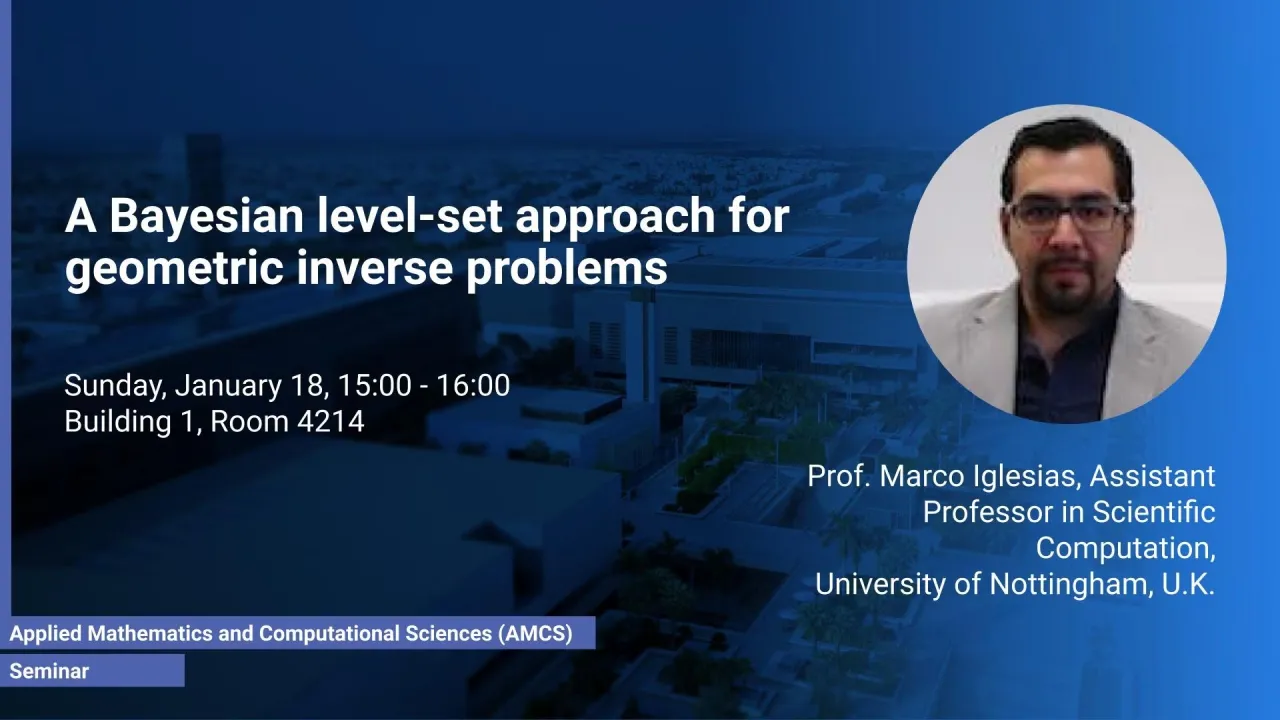
A Bayesian level-set approach for geometric inverse problems
Marco Iglesias received his PhD in 2008 from the Institute for Computational Engineering and Sciences, University of Texas at Austin. From 2008 and 2013 Iglesias held postdoctoral positions at the Department of Civil and Environmental Engineering at MIT as well as the Mathematics Institute at the University of Warwick . Since October 2013 Iglesias is an assistant professor in scientific computation at the University of Nottingham, U.K.
Overview
Abstract
A Bayesian level-set approach for geometric inverse problems
Joint work with Yulong Lu (Warwick) and Andrew Stuart (Warwick University)
We discuss a computational Bayesian framework for the solution of PDE-constrained inverse problems where the unknown is a geometric feature of the underlying PDE forward model. Geometric inverse problems of this type arise, for example, in the characterisation of subsurface formations where interfaces between geologic structures must be inferred from subsurface flow measurements. Another example emerges from Electrical Impedance Tomography (EIT) where the geometry of unhealthy tissue must be inferred from measurements of voltages associated to current distributions of a given configuration of electrodes. In this talk we introduce a level-set approach to infer and quantify the uncertainty of unknown geometric features in the aforementioned inverse problems within an infinite dimensional Bayesian framework.
We first discuss models in which the geometry is defined by means of a finite number of parameters, utilizing descriptions which are sufficiently general to include layered media, faults and channels, and to allow for spatial variability within the different components of the field .
We then proceed to demonstrate the application of Bayesian level-set formulations which allows for more complex geometric structures characterised by an infinite set of parameters .
Numerical experiments are displayed to show the capabilities of the level-set approach for (i) inverse source detection (ii) identification of geologic structures in groundwater flow (iii) estimation of electric conductivity in the complete electrode model for EIT. In these three applications, the unknown parameters have sharp interfaces characterised by the level-set function which is inferred, within the Bayesian framework, from observational data that arises from the PDE (forward) model under consideration. By means of state-of-the-art MCMC methods we describe numerical experiments which explore the posterior distribution that arises from the Bayesian level-set formulation.
M.A. Iglesias, K. Lin, A.M. Stuart, "Well-Posed Bayesian Geometric Inverse Problems Arising in Subsurface Flow", Inverse Problems 30 (2014) 114001. http://arxiv.org/abs/1401.5571
M.A. Iglesias, Y. Lu, A.M. Stuart, "A level-set approach to Bayesian geometric inverse problems", In Preparation, 2014.
Brief Biography
Marco Iglesias received his PhD in 2008 from the Institute for Computational Engineering and Sciences, University of Texas at Austin. From 2008 and 2013 Iglesias held postdoctoral positions at the Department of Civil and Environmental Engineering at MIT as well as the Mathematics Institute at the University of Warwick . Since October 2013 Iglesias is an assistant professor in scientific computation at the University of Nottingham, U.K. His main research interest is the solution of PDE-constrained inverse problems. In particular, Iglesias' area of expertise lies in the computational aspects of classical and Bayesian inverse problems that arises from large-scale applications.