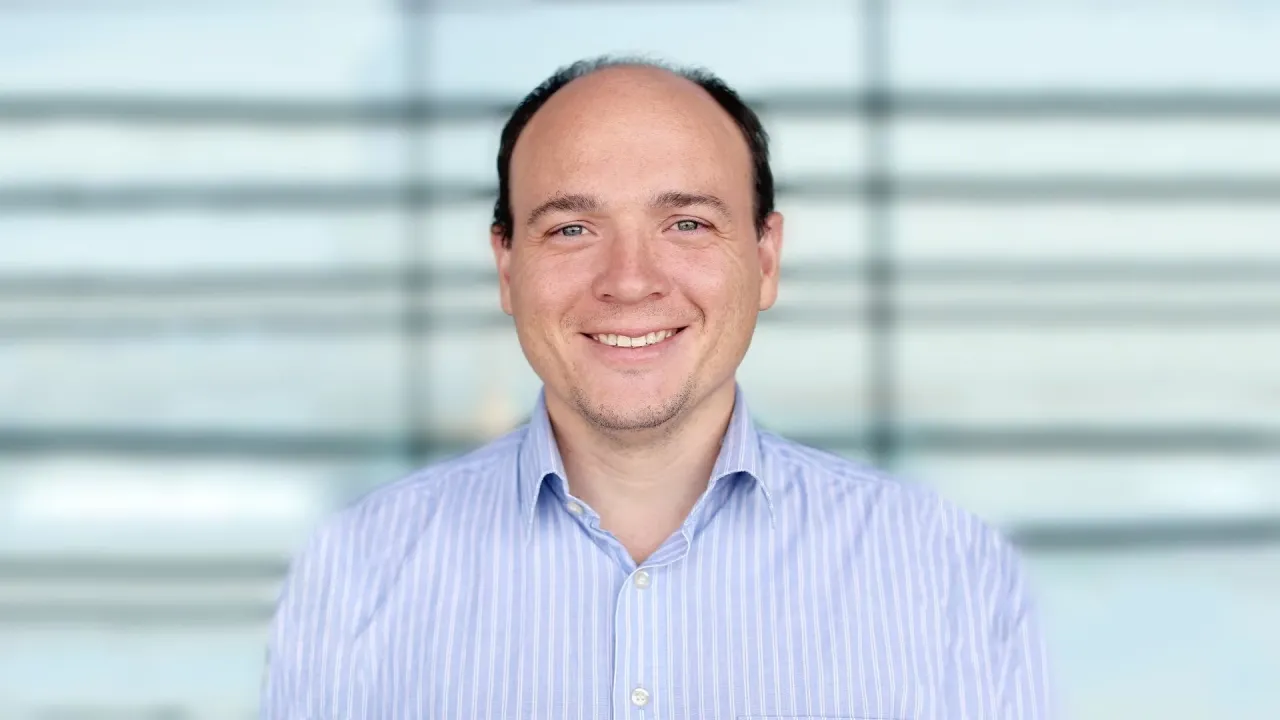
Hierarchical matrix introduction course by Dr. Alexander Litvinenko (KAUST) and Dr. Rio Yokota (KAUST)
Alexander Litvinenko joined the Stochastic Numerics Group and Strategic Initiative in Uncertainty Quantification at KAUST in 2013. He specializes in efficient numerical methods for stochastic PDEs, uncertainty quantification, and multi-linear algebra. He is involved in Bayesian update methods for solving inverse problems, with the goal of reducing the complexity of both the stochastic forward problem as well as the Bayesian update by a low-rank (sparse) tensor data approximation.
About
- Class schedule: Apr. 22nd and 23rd, 2014 from 04:00 pm to 06:30 pm
- Location: Computer Lab (the third floor in the library)
Biography
Alexander Litvinenko joined the Stochastic Numerics Group and Strategic Initiative in Uncertainty Quantification at KAUST in 2013. He specializes in efficient numerical methods for stochastic PDEs, uncertainty quantification, and multi-linear algebra. He is involved in Bayesian update methods for solving inverse problems, with the goal of reducing the complexity of both the stochastic forward problem as well as the Bayesian update by a low-rank (sparse) tensor data approximation. A further goal is the optimal design of experiments to maximize the information gain for a given amount of experimental effort. Applications include aerodynamics, subsurface flow, and climate prediction. Alexander earned B.S. (2000) and M.S. (2002) degrees in mathematics at Novosibirsk State University, and his Ph.D. (2006) in the group of Prof. Hackbusch at Max-Planck-Institut in Leipzig, on the combination of domain decomposition methods and hierarchical matrices for solving elliptic PDEs with jumping and oscillatory coefficients. From 2007-2013 he was a Postdoctoral Research Fellow at the TU Braunschweig in Germany where he became interested in his current research focus area of low-rank/sparse methods for uncertainty quantification as well as Bayesian updating for multi-parametric problems.