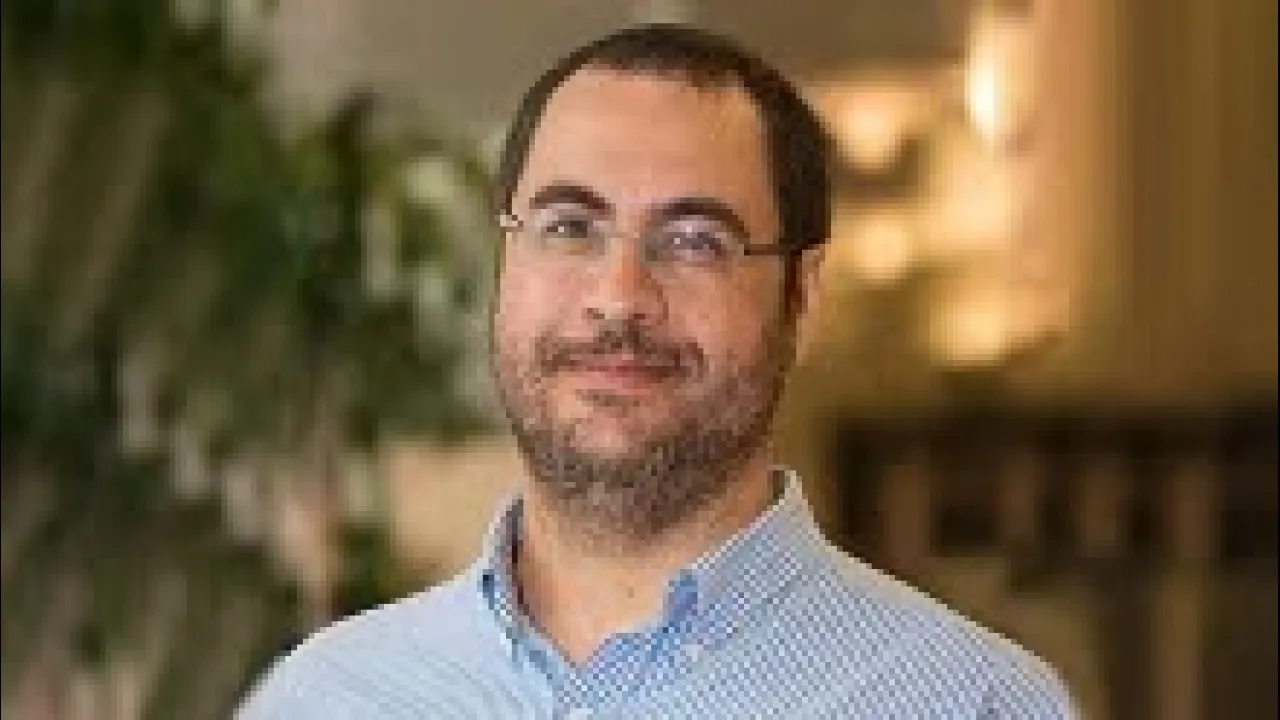
Alvaro Moraes successfully defended his PhD thesis
On Jan 26th, 2015, Alvaro Moraes successfully defended his Ph.D. thesis entitled “Simulation and Statistical Inference of Stochastic Reaction Networks with Applications to Epidemic Models”.
About
On Jan 26th, 2015, Alvaro Moraes successfully defended his Ph.D. thesis entitled “Simulation and Statistical Inference of Stochastic Reaction Networks with Applications to Epidemic Models”.
Supervisor: Prof. Raul Tempone (KAUST - KSA)
Committee Members: Prof. Omar Knio (KAUST - KSA)
Prof. Marc Genton (KAUST - KSA)
Prof. Fabrizio Bisetti (KAUST - KSA)
Prof. Boualem Djehiche (KTH - Sweden)
External Examiner: Prof. Mike Giles (University of Oxford, UK)
Abstract
Markovian epidemic models are stochastic models belonging to a wide class of pure jump processes known as Stochastic Reaction Networks (SRNs) that have been mainly developed to model biochemical reactions. SRNs also have applications in neural networks, virus kinetics, and dynamics of social networks, among others.
This Ph.D. thesis is focused on novel fast simulation algorithms and statistical inference methods for SRNs.
Our novel Multi-level Monte Carlo (MLMC) hybrid simulation algorithms provide accurate estimates of expected values of a given observable of SRNs at a prescribed final time. They are designed to control the global approximation error up to a user-selected accuracy and up to a certain confidence level, and with near-optimal computational work.
Regarding the statistical inference aspect, we first mention an innovative multi-scale approach, where we introduce a deterministic systematic way of using up-scaled likelihoods for parameter estimation while the statistical fittings are done in the base model through the use of the Master Equation. In a different approach, we derive a new forward-reverse representation for simulating stochastic bridges between consecutive observations. This allows us to use the well-known EM Algorithm to infer the reaction rates.
Related research:
Multiscale Inference for Pure Jump Processes
Multilevel Approximation of Stochastic Reaction Networks
The forward-reverse algorithm for stochastic reaction networks with applications to statistical inference