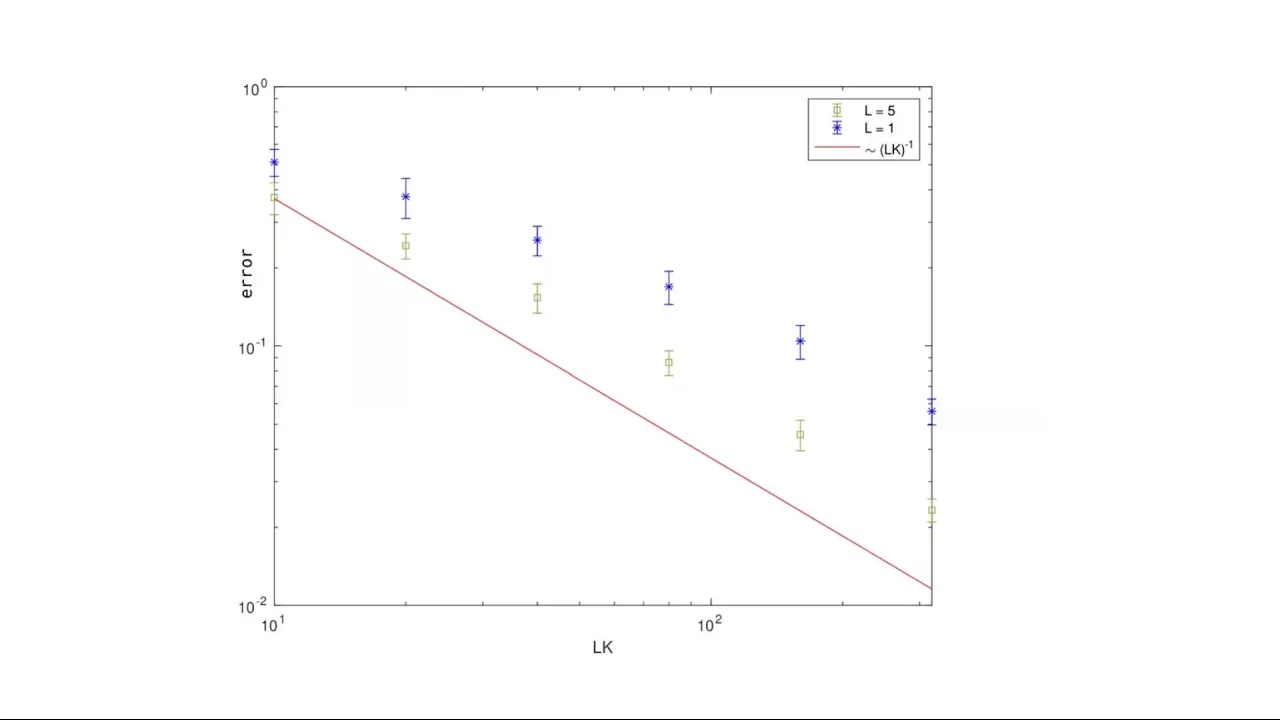
Article published in IMA Journal of Numerical Analysis
About
In September 2022, the IMA Journal of Numerical Analysis published the article Smaller generalization error derived for a deep residual neural network compared with shallow networks, by Aku Kammonen (KAUST), Jonas Kiessling (KTH Royal Institute of Technology), Petr Plecháč (University of Delaware), Mattias Sandberg (KTH Royal Institute of Technology), Anders Szepessy (KTH Royal Institute of Technology), and Raul Tempone (KAUST).
Abstract: Estimates of the generalization error are proved for a residual neural network with L random Fourier features layers. An optimal distribution for the frequencies of the random Fourier features is derived. This derivation is based on the corresponding generalization error for the approximation of the function values f(x). The generalization error turns out to be smaller than the estimate of the generalization error for random Fourier features, with one hidden layer and the same total number of nodes KL, in the case of the L∞-norm of f is much less than the L1-norm of its Fourier transform f̂ . This understanding of an optimal distribution for random features is used to construct a new training method for a deep residual network. Promising performance of the proposed new algorithm is demonstrated in computational experiments.